We are entering an AI world where start-ups are transforming everyday lives through artificial intelligence and machine learning. AWS’ Allie K Miller spoke at the recent ScaleUp:AI conference and set out the key trends for ML and AI.
As artificial intelligence and machine learning have become more mainstream in the last decade; the tech sector has seen countless start-ups popping out of the ground.
From airport security to unlocking your smartphone to recommendations in retail and chatbot solutions, AI is becoming an increasingly influential presence in everyday life. For businesses, it is a catalyst in transformation, allowing for automation of operations and monetisation of data.
Statistically, in 2021, AI saw $66.8 billion in funding, which is double the amount seen in 2020 according to AWS global head of machine learning business development for start-ups and venture capital Allie Miller. Unicorn ‘births’ in the sector went from 12 to 65.
Speaking at the ScaleUp:AI conference last week, Miller credited this boom to “the realisation of the business value of artificial intelligence.”
With her team, Miller works with start-ups to help them raise $100 million and get acquired, and part of her job “is to have a crystal ball to the future of AI.”
“We’re thinking three to five years ahead of time to figure out what technologies are really picking up speed,” she told the conference in New York. And with that knowledge, Miller shared what trends she sees on the horizon for machine learning and artificial intelligence.
Where is AI now?
For Miller, AI and its use can be summarised within ‘the three P’s’: Personalisation, prediction, and proactiveness.
For personalisation, every single thing becomes a market of one whether that is a customer, company, or maybe even a whole country. This means AI will have an algorithm for a customer’s grocery shopping to figure out when to buy avocados and a healthcare algorithm that tells you how to get in the best shape possible.
Prediction, the second P, forecasts activity before it happens. So, essentially the AI will know when a specific person is expected to want a new pair of socks, and it will also know when an elevator is about to have a malfunction or broken component.
This prediction power means nothing if you don’t take advantage of it, Miller said, and that is where “proactive” comes in. Proactive AI will ship the socks to the customer before they’ve bought them, and will also order the new component for the elevator, and put in the work order to get someone to fix it before it breaks down.
Today, AI systems can perform customer scoring, such as approving mortgages and credit cards. It can translate 71 languages. AI can also forecast extremely well – Miller says that non-ML implementations are extremely brittle when it comes to unpredictable events, such as the sudden arrival of Covid-19. AI can also be used to find operational efficiencies such as fraud detection, identification, verification, and face similarity comparison – something that you’d see at passport control in airports. Chatbots are a classic AI use case, and they are only getting smarter. Finally remote working has meant for more speech-to-text (RPA voice), collaboration and audio-visual automatic speech recognition such as the journalist’s favourite Otter AI.
What is on the horizon for AI and ML?
To stay on top of machine learning trends Miller recommends looking at what start-ups are doing, as well as regulators, and researching the latest ML papers.
Whilst she believes there are many trends incoming for the future of ML and AI, she breaks it down to low data, security, decentralisation, and increase in access.
Low Data
Supported by recent language models (a tool that predicts words such as predictive text and translation), low code or no-code platforms (platforms that rely on visual programming for workflows, data visualisation, and designing, for example) are becoming more when developing applications.
Miller said the number one thing she expects to see is a new generation of massive transformer language models or GTP-3 examples. What this means is an increase in language translators and predictive text that goes above and beyond the spoken language – such as code translation.
Miller and her team see these large language models as a new platform that will shift how software, AI, and content will be created. According to Miller, start-ups within this sector are the fastest hitting unicorn status.
Also rising within the low data space is synthetic data – data that’s artificially manufactured rather than generated by real-world events. Miller pointed to face-ID, which is used to unlock phones, as an example as it uses synthetic data to recognise the user from a different angle, or with sunglasses on or a mask.
Security
Miller expects organisations to leverage machine learning for compliance and bias mitigation in the usage, processing and storage of personal data.
In industries such as healthcare and finance, where the transfer, extraction, and deletion of data is heavily regulated, start-ups such as Gretel.AI will step in to offer synthetic data. By using AI, Gretel.AI offers companies anonymised results without having to jump through regulation hoops while still meeting data protection laws.
This technique, known as neural search, will be offered to regulated markets, such as healthcare, finance and even governments, and offers an internal, encrypted search engine for these industry’s firms so that they can use data without worrying about users’ privacy and regulations.
Gretel.Ai has already raised almost $70 million working on this privacy as a service.
According to Miller, access extraction, purpose limitations, usage, storage, transference and deletion of data is a huge space and start-ups are only choosing one of these nouns to grow a company from, and are building over $100 million from it.
Decentralisation
Decentralisation for AI derives from the growth of individually owned data. Currently, many people already own their own healthcare data through wearables (heart rate, glucose levels etc.), but Millers says we’re going to see a rise in individually owned data in other things like automotive, retail, and transaction data.
“This growth of industry-owned data is ushering in a new wave of data and AI needs,” she says. “Technology like federated learning needs to come to be able to manage this process.”
Federated learning is an AI technique that helps train machine learning models without sending sensitive user data to the cloud.
“Decentralisation of ML essentially means distributing the training of neural networks or ML models across a cluster of machines by partitioning the data and the model.”
Increase in access
Finally, Miller recognises that, currently, even the largest organisations are struggling to find ML talent.
Skills gaps in data, systems, and security are common and, statistically, demand for AI specialists has gone up by 74% and for data specialists, the demand has gone up by 37%.
Notably, “when you look at job postings and LinkedIn data, there is three times the amount of AI and machine learning jobs” as compared to people searching for a job in this sector.
“This is a massive and growing gap and we don’t yet have the skillset to be able to complete that.”
Miller said that while these large organisations are investing in upskilling, it’s not just upskilling that’s needed it’s also reskilling, with easier tools and frameworks another key helping point such as low code platforms.
Written by Nicole Deslandes and republished with permission from TechInformed.
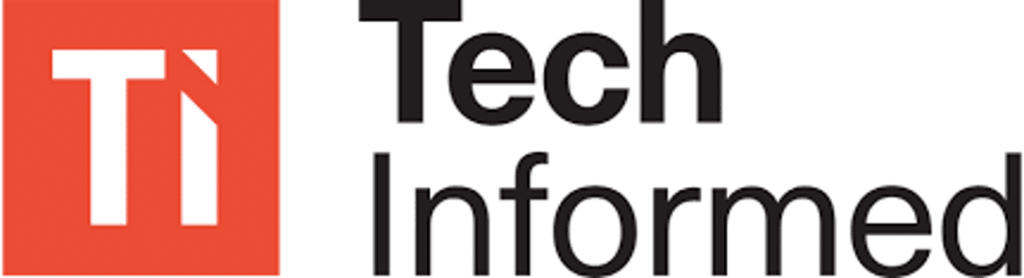